MetaboPredict
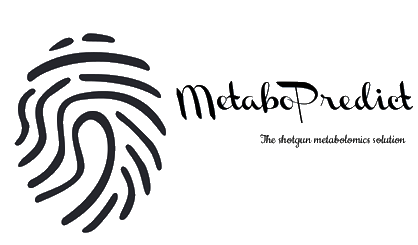
The metabolomic approach can be defined as "targeted" or "untargeted", depending on whether or not it is guided by an a priori hypothesis. The first is a highly specific approach that focuses on the qualitative and quantitative analysis of a predetermined set of metabolites; the second, starts from the evaluation of the greatest number of metabolites possible, for the definition of metabolic patterns associated with a given condition. Therefore, the primary purpose is not the identification of single (or groups of) metabolites, but rather the definition of the metabolic characteristics that are able to discriminate between the analyzed groups . In other words, with this approach, we focus on the analysis of the relationships that metabolites establish between them. The approach not guided by a priori hypotheses has the advantage of identifying unexpected or non-trivial biomarkers that allow a better understanding of pathophysiological mechanisms.
The large amount of data obtained from the analysis of the metabolome and the consequent process of extracting information from these data requires the definition of a pipeline for the management of the untargeted approach, with the aim of providing a quick, simple and usable tool. for the "data analysis" step.
MetaboPredict© represents the solution to this need. By means of this software it is in fact possible to carry out the global study of metabolites and to predict the most probable class of belonging of unknown samples. By means of this application it is possible both to perform the exploratory analysis steps and to structure and train supervised classification algorithms which can then be used as independent classifiers or combined in a single "ensemble" model.
SIMPLE INTERFACE AND INTUITIVE PIPELINE
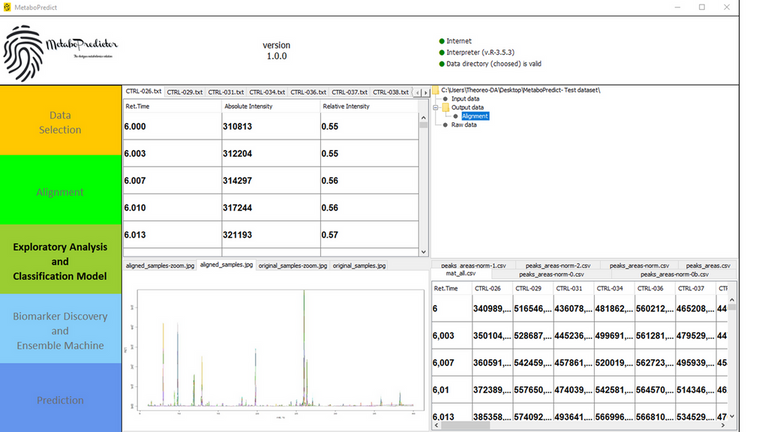
MetaboPredict has been designed to show the user a simple and clean graphical interface. The data analysis is guided by means of a very intuitive and complete pre-established pipeline that allows you to perform a broad exploratory analysis and to train different machine learning models, both supervised and unsupervised. The results shown in both graphical and tabular form are also saved as separate files for use in other surveys or by means of other data analysis or graphics software.
MetaboPredict also allows you to enclose the models developed in an "Ensemble" classifier that can also be used for the prediction of unknown samples or to validate the results obtained by means of independent datasets.
GUIDED BUT ALWAYS CUSTOMIZABLE ANALYSIS
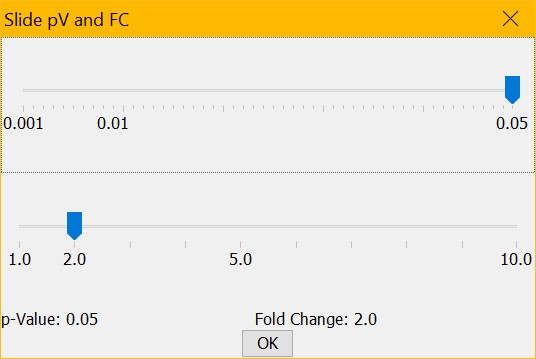
Although MetaboPredict guides the user step by step for the management of data deriving from metabolomics investigations, all the settings are highly customizable and different cut-offs can be set to direct the analysis and narrow or broaden the analysis criteria. Default settings are proposed but these can be changed in a very simple and intuitive way.
INTERACTIVE 3D GRAPHICS
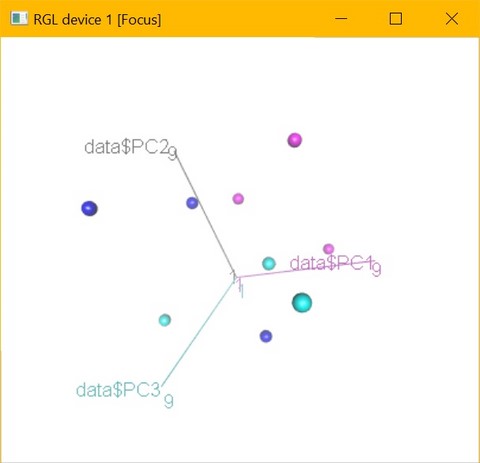
The results can also be viewed by means of interactive 3D graphics that are saved as html files. These can therefore be easily visualized and rotated by means of any web browser. This type of visualization offers the possibility to explore the data from different angles, letting the relationship between the objects shine through in a very simple way.
EFFICIENT MANAGEMENT OF MULTI-CLASS PROBLEMS
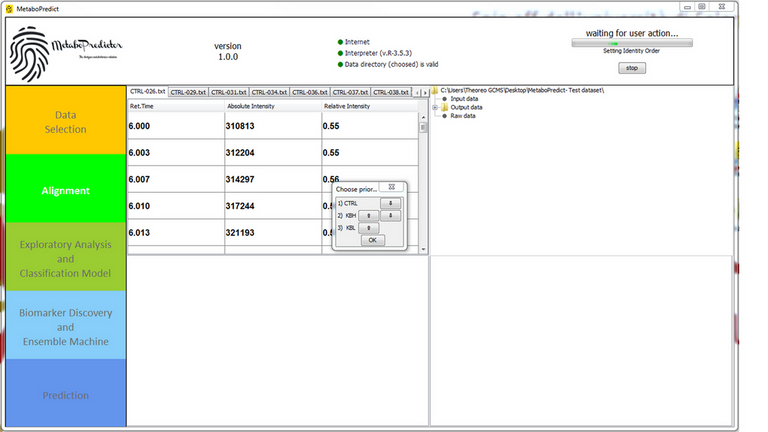
MetaboPredict is able to manage multiclass tasks. Nevertheless, in addition to traditional management, it proposes a subdivision of these problems into a series of tasks on a dichotomous basis, offering a customizable hierarchy in order to privilege some aspects of the phenomenon studied.
This approach has proved particularly useful for managing multiclass tasks by means of supervised machine learning algorithms which are particularly efficient for handling Boolean problems.